Unlocking the Power of Data Annotation Tools and Platforms
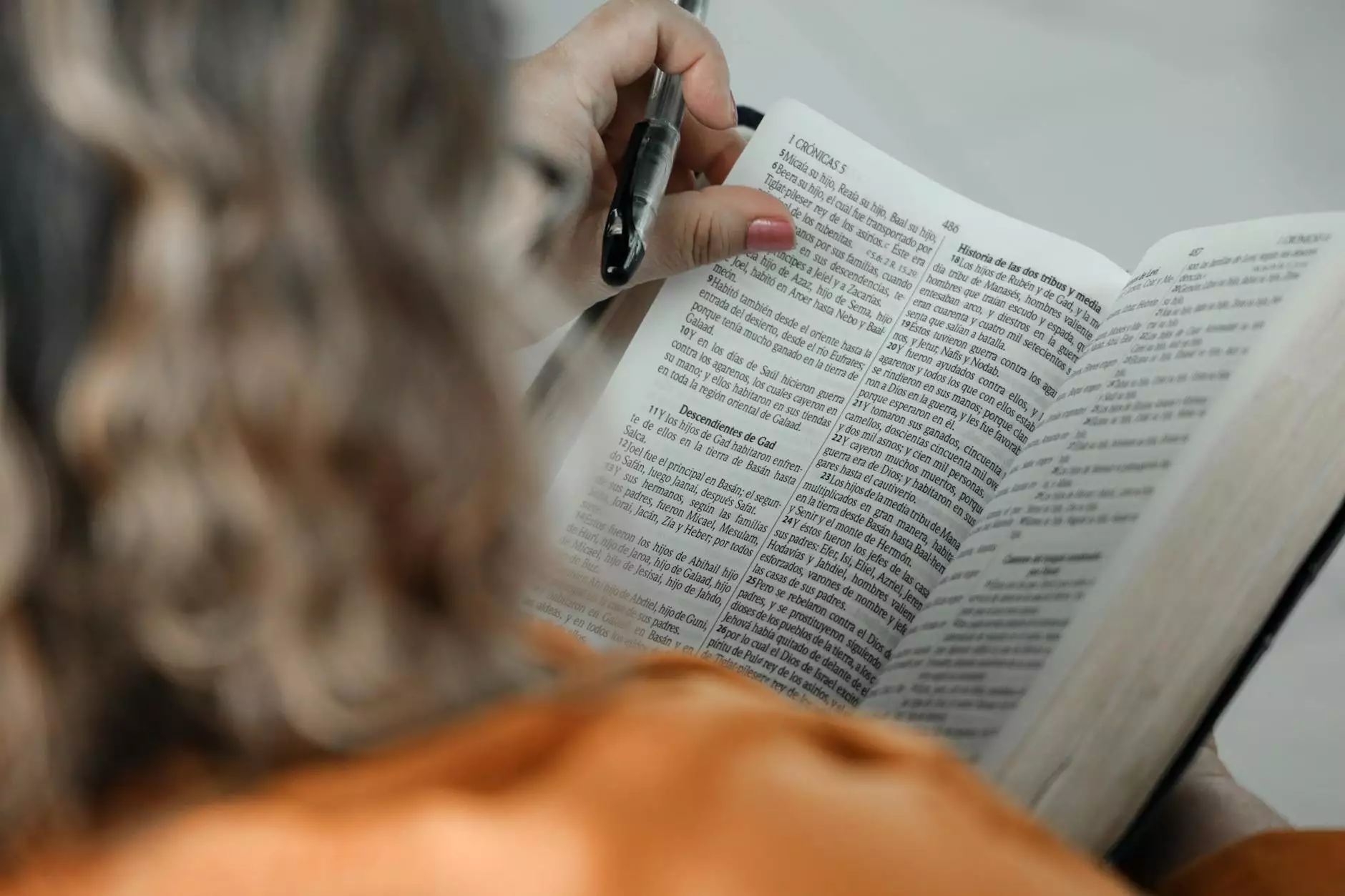
In the world of artificial intelligence, machine learning relies heavily on accurate data processing. One of the key components in achieving precise machine learning outcomes is through effective data annotation. Data annotation tools and platforms play a pivotal role in refining the datasets, ultimately empowering AI models to make informed decisions. This article dives deep into the importance of these tools, focusing on the advanced solutions provided by keylabs.ai.
Understanding Data Annotation
Data annotation refers to the process of labeling or tagging data so that machine learning algorithms can interpret it correctly. This crucial step transforms raw data into a structured format that AI systems can understand and learn from. Without data annotation, machine learning models would struggle to find patterns and make predictions.
The Significance of Data Annotation in AI
Data annotation serves multiple purposes within the realm of artificial intelligence:
- Enhancing Accuracy: Properly annotated data significantly increases the performance accuracy of machine learning models. It reduces the likelihood of errors, ensuring that the models produce reliable outputs.
- Aiding in Classification: Different types of annotating help in classifying data correctly, such as object detection in images or sentiment analysis in text.
- Supporting Diverse Applications: Data annotation is essential in various industries, including healthcare, automotive, and finance, paving the way for innovative applications like autonomous driving and personalized medicine.
Categories of Data Annotation Tools
Data annotation tools can be categorized based on their functionalities and applications. Here are some common types:
1. Image Annotation Tools
Image annotation tools are designed to label images for computer vision tasks. They enable the identification and classification of objects within images. Common annotation techniques include:
- Bounding Boxes: Enclosing an object with a rectangle.
- Polygon Segmentation: Defining the outline of an object accurately.
- Keypoint Annotation: Marking specific points on an object or human landmark.
2. Text Annotation Tools
Text annotation tools assist in labeling textual data for tasks such as sentiment analysis and named entity recognition. Key techniques in text annotation consist of:
- Entity Recognition: Identifying and classifying key entities within the text.
- Sentiment Labeling: Determining the sentiment expressed in a given text.
- Part-of-Speech Tagging: Labeling words based on their grammatical functions.
3. Audio Annotation Tools
Audio annotation tools are used in the processing of sound data, crucial for speech recognition and audio classification. Specific techniques include:
- Transcription: Converting spoken words into text format.
- Sound Tagging: Identifying specific sounds within an audio clip.
- Emotion Detection: Recognizing emotions based on tone and pitch.
Choosing the Right Data Annotation Platform
Selecting the right data annotation platform is essential for optimizing the data labeling process. Here are some critical factors to consider:
- User-Friendliness: The platform should have an intuitive interface that allows users to easily navigate and perform annotations.
- Scalability: Ensure that the platform can handle varying amounts of data as your project grows.
- Collaboration Features: A good annotation platform facilitates teamwork, enabling multiple annotators to work seamlessly.
- Quality Assurance: Look for platforms that offer quality control measures to uphold annotation accuracy.
- Integration Capabilities: Check if the platform can integrate with your existing workflows and tools.
Keylabs.ai: Leading the Way in Data Annotation Solutions
Among the plethora of data annotation tools and platforms available, keylabs.ai stands out for its innovative approach to data labeling. The platform provides comprehensive solutions that cater to various industries and applications.
Why Choose Keylabs.ai?
Keylabs.ai offers a rich set of features that enhance the data annotation process, including:
1. Advanced Annotation Techniques
The platform supports multiple annotation forms, ensuring versatility across different data types— be it images, text, or audio. Its capabilities provide the flexibility needed for varied machine learning projects.
2. Automated Annotation Tools
Utilizing cutting-edge AI technologies, Keylabs.ai automates repetitive tasks, significantly reducing the time spent on data annotation. Automation not only speeds up the process but also minimizes human error.
3. Robust Quality Assurance Protocols
The platform employs rigorous quality assurance measures to ensure the integrity of the annotated data. This includes review processes and feedback loops, which enhance the quality and reliability of the annotations.
4. Seamless Collaboration
Keylabs.ai promotes collaborative work, allowing teams to annotate data together. This feature aids in maintaining consistency and efficiency within teams of different sizes.
5. Comprehensive Support and Training
Understanding the importance of user experience, Keylabs.ai provides extensive support and training to users, ensuring that they can leverage the platform’s capabilities to the fullest.
Case Studies: Success Stories with Keylabs.ai
To illustrate the effectiveness of Keylabs.ai, let’s examine a few success stories:
Case Study 1: Enhancing Healthcare Analytics
A leading healthcare provider utilized Keylabs.ai to annotate medical images for a diagnostic tool. By employing advanced image annotation techniques, the healthcare provider improved diagnostic accuracy significantly, leading to better patient outcomes.
Case Study 2: Autonomous Vehicle Development
An autonomous vehicle tech company leveraged Keylabs.ai's platform to tag thousands of images collected from real-world driving scenarios. This extensive image dataset enabled them to train robust AI models, resulting in safer and more reliable self-driving technologies.
Case Study 3: Elevating Customer Engagement in Retail
A major retail brand used Keylabs.ai to annotate customer interaction data, allowing them to understand customer sentiment and behavior better. The insights derived through annotated data helped in tailoring marketing strategies, enhancing customer engagement by 40%.
The Future of Data Annotation
As the demand for AI-powered applications continues to grow, the need for advanced data annotation tools will escalate. With the rise of automation and machine learning, platforms like keylabs.ai will play a crucial role in shaping the future of data processing. Here are several trends to watch for:
- Increased Automation: The continued advancement of machine learning algorithms will increasingly allow for more automated annotation processes.
- Integration with AI Technologies: Future data annotation tools will likely harness AI to predict and enhance annotation accuracy.
- Focus on Ethics and Data Privacy: With growing concerns about data privacy, future platforms will need to prioritize ethical standards and secure data handling practices.
Conclusion
In conclusion, data annotation is an essential pillar of machine learning and artificial intelligence. The strategic use of advanced data annotation tools and platforms, such as keylabs.ai, can greatly enhance the quality of data, leading to more accurate and effective AI systems. As businesses continue to harness the power of AI, investing in robust data annotation solutions will be pivotal for their success in the competitive landscape.
https://keylabs.ai/